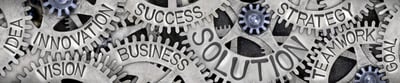
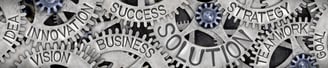
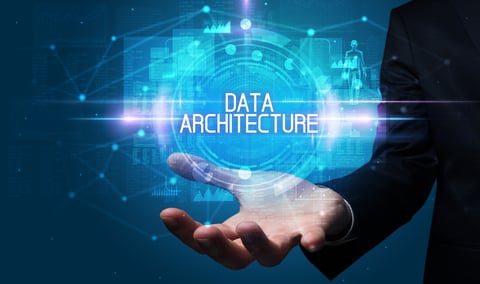
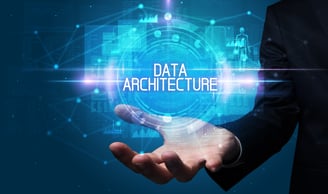
Data Architecture and Strategy
Enterprise Architecture
Enterprise Architecture (EA) involves creating a strategic framework that aligns an organization’s business objectives with IT infrastructure. It encompasses the design, implementation, and management of technology solutions to ensure seamless integration and operation of business processes. EA aims to enhance organizational agility, efficiency, and effectiveness by providing a structured approach to managing and utilizing IT resources.
Data Architecture
Data architecture is a subset of enterprise architecture that focuses on the organization's data assets, data management processes, and the technologies used to manage data. It involves designing and maintaining the data environment to ensure data is accurately collected, stored, and utilized for business decision-making.
Core Functions of Data Architecture
Framework Development:
Define data architecture frameworks, standards, and principles.
Establish data models, metadata, security protocols, and reference/master data management.
Data Management:
Plan, specify, enable, create, acquire, maintain, use, archive, retrieve, control, and purge data.
Ensure data is managed effectively throughout its lifecycle.
Integration and Coordination:
Collaborate with various departments, stakeholders, and external vendors to ensure data flows are consistent and reliable.
Define data flows, identifying which parts of the organization generate and use data, and how data transitions are managed.
Reference Architecture:
Develop reference architectures that provide patterns for creating and improving data systems.
Technology Alignment:
Translate business requirements into technical specifications, including data streams, integrations, transformations, databases, and data warehouses.
Align data architecture with enterprise strategy and related business architecture.
Evolving Data Architectures
Data architecture has evolved from managing structured transaction data in relational databases to incorporating unstructured and semi-structured data through big data technologies. Modern architectures often include data lakes and data lakehouses, which combine elements of traditional data warehouses and big data storage, enabling real-time data processing and advanced analytics applications.
Best Practices for Data Architecture
Solid Data Foundation:
Create robust data models representing business processes.
Design schemas mapping relationships between data entities and optimize database architecture for efficient data handling.
Data Observability:
Continuously monitor data health using tools that identify and resolve data quality issues.
Data Cataloging:
Implement data catalogs for metadata management, data discovery, and collaboration, ensuring easy access and understanding of data.
Data Governance:
Establish and enforce data governance policies to ensure data consistency, quality, and compliance with regulations.
Conduct regular data audits to maintain transparency and accountability.
Data Security:
Implement robust security measures including encryption, multi-factor authentication, and regular backups to protect data from breaches and cyber threats.
Data Validation and Cleansing:
Ensure data accuracy and consistency through validation, normalization, and cleansing processes.
Scaling Data Analytics
Scaling data analytics involves fostering a data-driven culture, integrating analytics with other enterprise systems, and advancing into predictive analytics and machine learning. It requires selecting appropriate tools for data storage, ETL (Extract, Transform, Load), and machine learning to manage and analyze data effectively.
For a comprehensive and efficient solution, consider exploring alternatives with us.
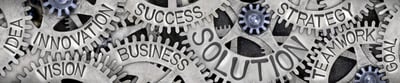
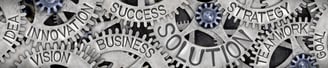
CONTACT
PHONE
Office: (682) 215-6860
Emergency: (817) 300-0868
EMAIL
contact@coker-business-solutions.com
coker.karen@coker-business-solutions.com
LOCATION
DALLAS/FORT WORTH METROPLEX